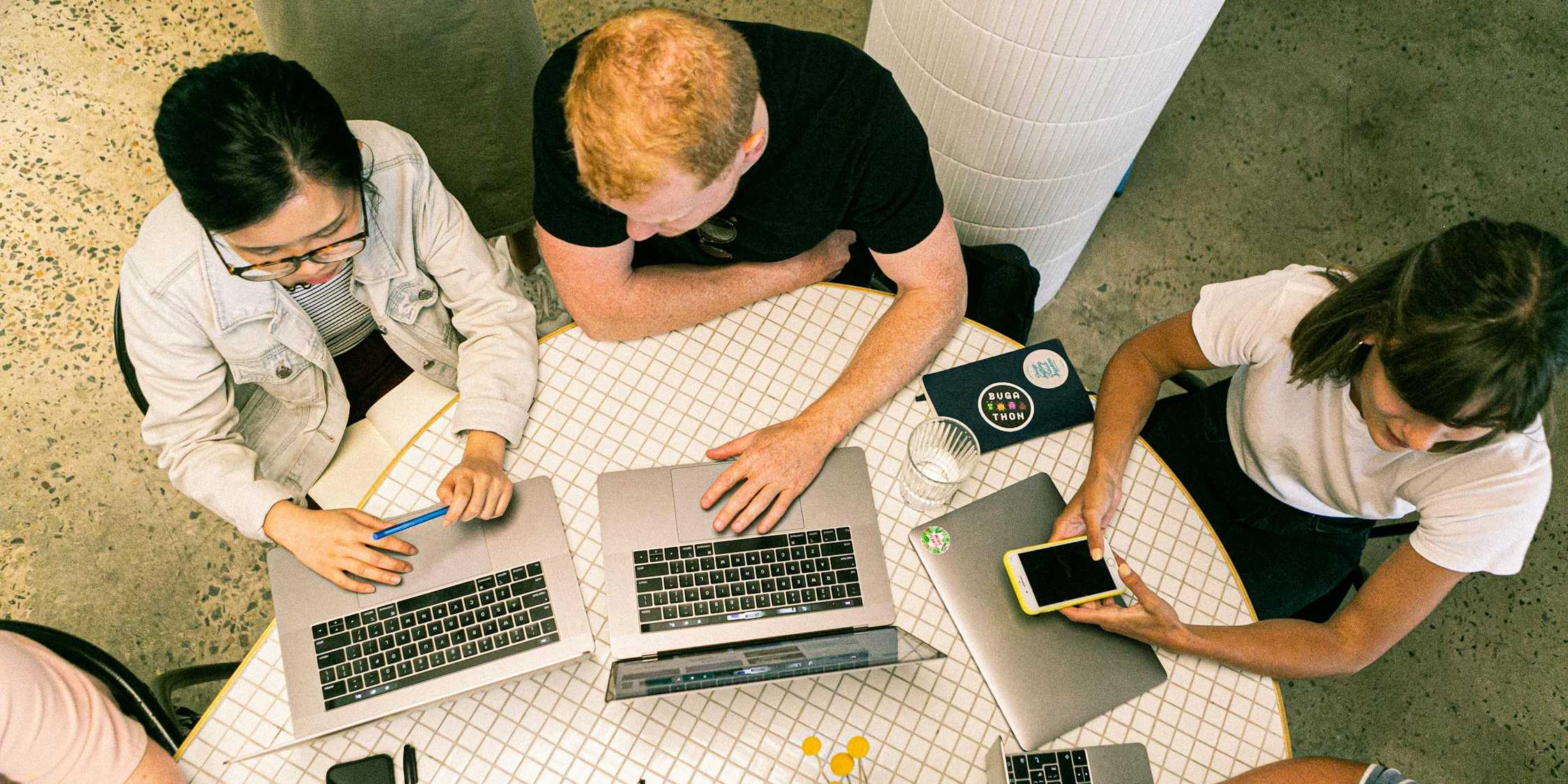
Harnessing Machine Learning for Systematic Reviews: A Case Study on Attrition in Conversational Agent-Delivered Mental Health Interventions
Ahmad Ishqi Jabir, PhD researcher at Future Health Technologies (FHT) programme shares his experience using ASReview Lab, an open-source machine learning tool, in his latest published study.
In Brief:
- Ahmad’s use of an open source machine learning tool, ASReview, in his latest study highlights its potential as a valuable tool for researchers to effectively manage large volumes of research publications to conduct systematic reviews.
- ASReview significantly reduces the time needed for the title and abstract screening step in systematic reviews by prioritising and sorting relevant studies.
- Despite its efficiency, human intervention is still required to guide the initial review process and ensure accuracy of selected papers.
Ploughing Through a Haystack: The Challenge of Screening Extensive Research Publications for Systematic Reviews
In the early stages of academic research, a major challenge researchers encounter is managing and screening the vast amount of existing scientific studies for systematic reviews. Systematic review is usually a methodical and comprehensive overview of focused research topics and conducting one involves searching databases, and then indentification and synthesis of relevant publications.
Yet, for research domains with an exponential influx of publications, sifting through thousands of papers for relevant ones can feel like searching for a needle in a haystack.
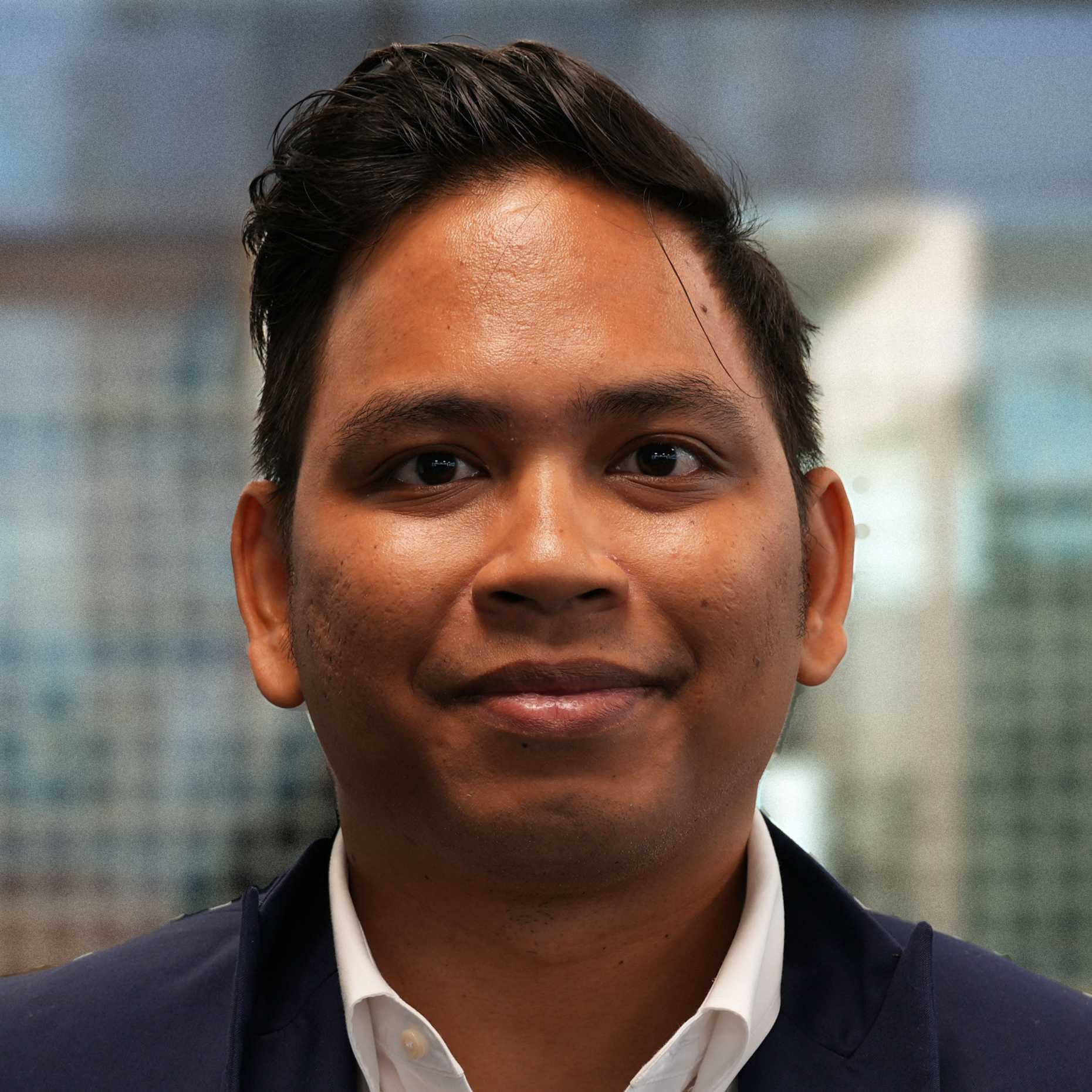
“It may take anywhere between 1 to 2 weeks to complete abstract and title screening for a team of 4 researchers, if we only focus on screening all day.”Ahmad Ishqi Jabir![]()
Ahmad further shared that the process was laborious and requires at least 2 people to dedicate their full attention and focus to this task alone to ensure accuracy.
ASReview: A Supplement to Enhancing Efficiency in Conducting Systematic Reviews
In a recent study conducted with researchers from external page Nanyang Technological University (NTU) and external page Monash University, Ahmad and his team sought to identify factors contributing to high attrition rates in mental health interventions delivered by conversational agents (CAs) or chatbots, with the goal of improving future clinical trials.
Approximately 4,000 titles and abstracts were reviewed with lesser manpower involved - how was this achieved?
Ahmad found a valuable ally in external page ASReview, an open-source machine learning tool which significantly sped up the reviewing process. What used to take 4 researchers weeks was now a one-week endeavour for him alone, with him dedicating only a few hours daily to reviewing and having more time to focus on other research.
How does ASReview work and perform?
Despite the substantial reduction in time, it is crucial to caveat that human intervention remains essential when using ASReview. After textual data (i.e, papers, manuscripts) are uploaded on it, Ahmad explains that the onus still lies on him to initially review the top papers of the digital stack and decide which fit the set research scope and parameters.
With multiple machine learning models within it, ASReview learns from his decision-making processes such as analysing keywords and themes of the included papers to actively reshuffle and prioritise the stack for his reviewing.
Other advantages of the tool include its capability to support a range of file formats and tabular data sets. Its versatility extends to running on personal computers as well as self-hosted local and remote servers, which helps ensure data ownership and confidentiality.
When asked about the precision of the tool, Ahmad shared that ASReview can provide better accuracy of screening performance, when compared to standard practices.
“But of course, as with other machine learning tools out there, ASReview is not a flawless tool. Its creators are still continuously improving and upgrading the tool. Being an open-source platform, users like me can help develop code extensions, contribute suggestions to the reviewing algorithm and more.”Ahmad Ishqi Jabir
As Ahmad also points out, resources on the ASReview Academy such as community discussion forums or online courses can be very helpful for users at any level of expertise.
Ahmad's experience exemplifies how integration of machine learning tools like ASReview into a study's systematic review process can be a form of support for researchers to manage the ever-expanding volume of research. In his own words, ASReview was a trusty 'co-pilot' which functioned as a safety net for relevant studies he could have missed after learning and adapting to his decision-making, leading to enhanced research efficiency and productivity.
Following his positive experience with ASReview, Ahmad has also encouraged fellow researchers in his research programme to adopt the tool for other research purposes. ASReview has since been used to review mobile mental health applications and short-list readings for an undergraduate course.
Jabir, A., Lin, X., Martinengo, L., Sharp, G., Theng, Y. and Tudor, Car L. (2024).
Attrition in Conversational Agent–Delivered Mental Health Interventions: Systematic Review and Meta-Analysis. J Med Internet Res, 2024.;26:e48168., doi: external page 10.2196/48168